- Core Technology
- Based on the foundation of proprietary technologies and SenseCore AI infrastructure,
SenseTime has rapidly opened up AI application in multiple vertical scenarios, and is empowering various industries.
- 01Game Agent
- 02League Learning
- 03DI-zoo Decision AI algorithm Zoo
- 04Autonomous Driving Decision System
- 05Traffic signal control
- 06Complex computational graph optimization
- 07Multimodal data processing
- 08DRL algorithm framework design
- 09Distributed DRL system design and optimization
01 / 09
Game Agent
We support artificial intelligence agents capable of accompanying, taking over offline players, high-level competing in real-time strategy, frist person shooting and sports games.
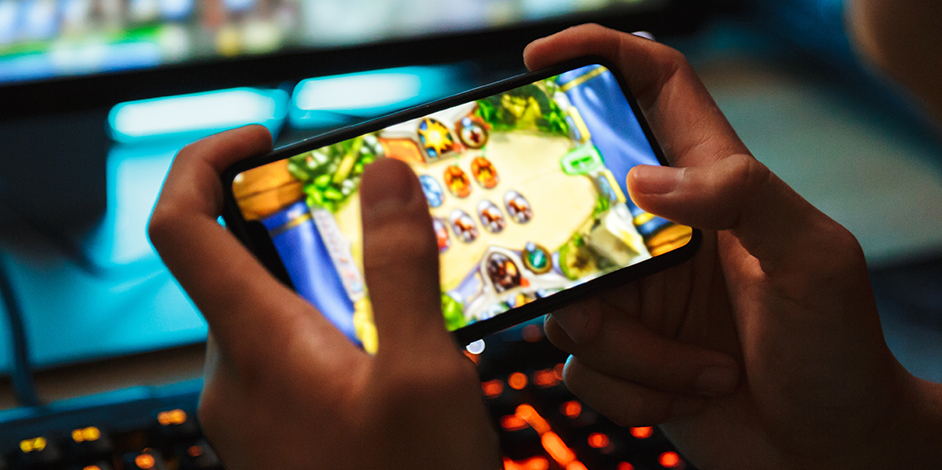
02 / 09
League Learning
Large-scale reinforcement learning framework. Starting from self-play, a single agent can expand to a league with hundreds of agents during training. This framework produces agents in different levels through well-designed match mechanism and massive games.
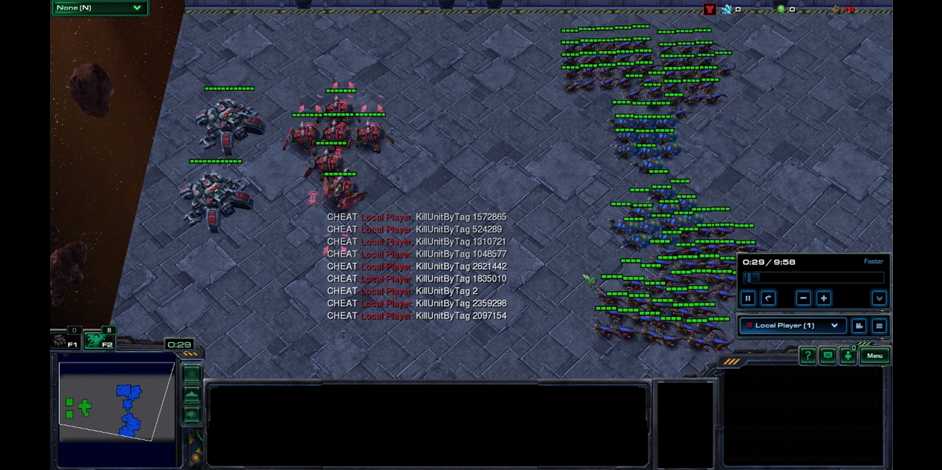
03 / 09
DI-zoo Decision AI algorithm Zoo
DI-zoo provides abundant implementations of reinforcement learning(RL) algorithms. It features well-tuned training configurations, efficient implementations and a pretrained algorithm zoo. We aim at helping researchers and engineers to quickly start learning RL, validate their ideas and easily get baselines for their custom environments.
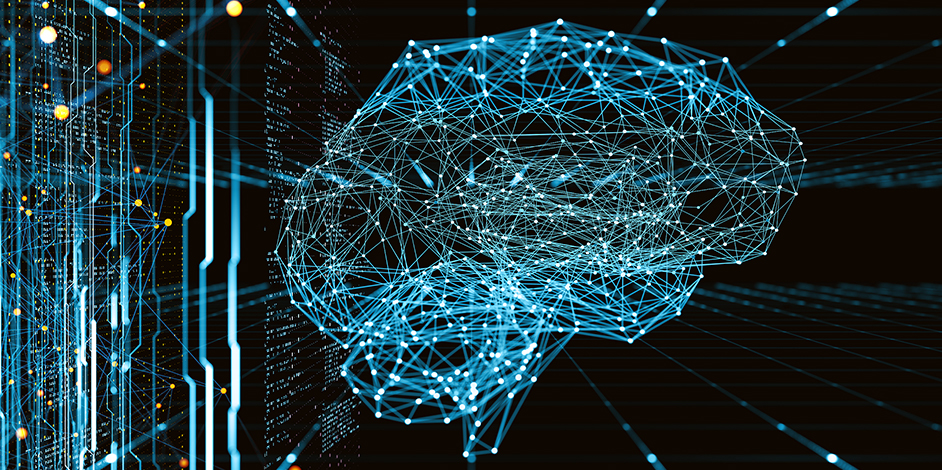
04 / 09
Autonomous Driving Decision System
Build an essential bridge between Decision AI and Autonomous Driving. This system helps to apply advanced Decision AI algorithms in academia to auto-driving tasks, and conduct training as well as evaluation with real vehicle data.
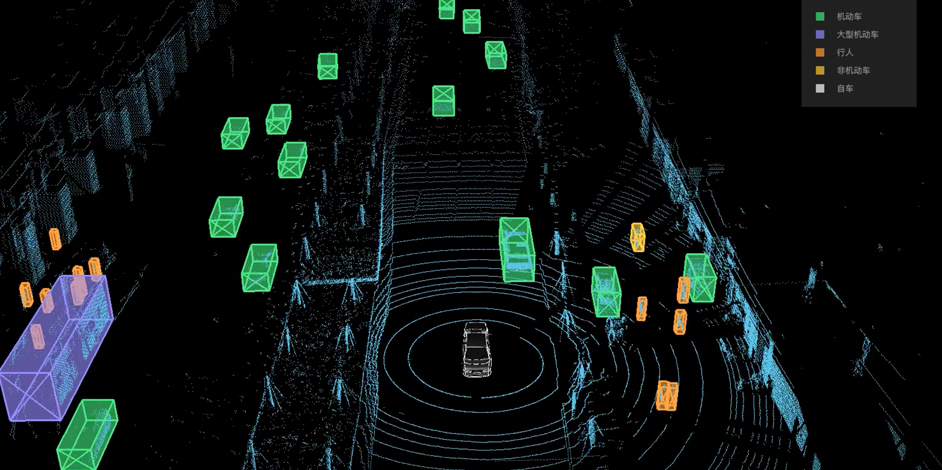
05 / 09
Traffic signal control
The Decision Intelligence technology is applied to city-level traffic signal light control, tidal lane change, etc., to maximize transportation efficiency. The actual effect on-road tests is 8%~47% higher than that of the traditional signal control methods.
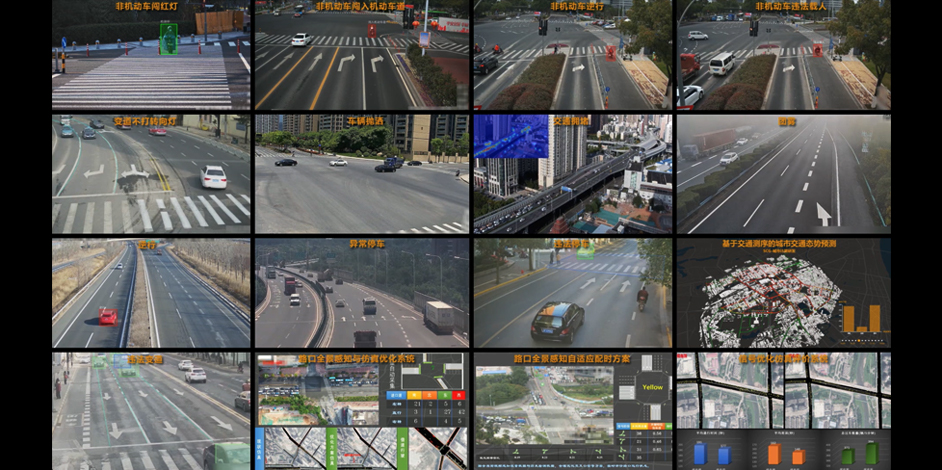
06 / 09
Complex computational graph optimization
According to the data flow optimization, design of lightweight computational graph and stable optimization of large-scale model training, it realizes the training optimization of the complex computational graph with high performance and low resource cost.
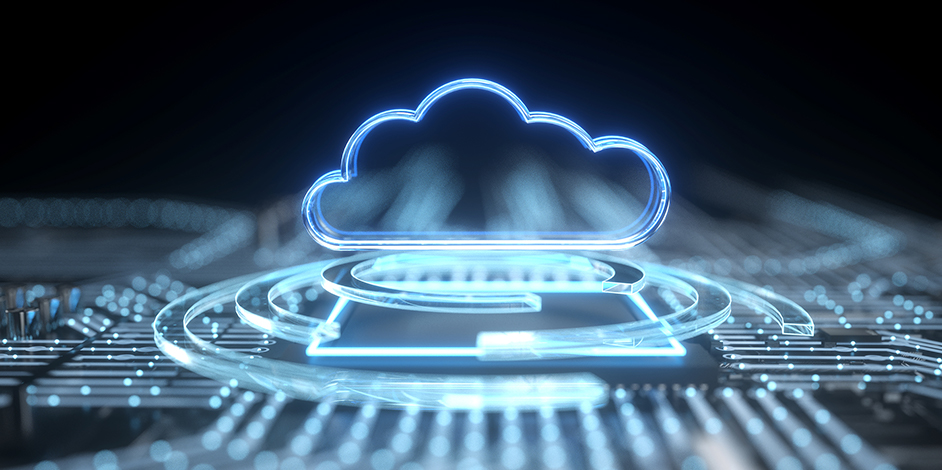
07 / 09
Multimodal data processing
According to the design of complex information extraction architecture for different modal data, it quickly realizes the effective feature extraction and efficient information aggregation of multiple modal data, and empowers model optimization based on multi-modal information.
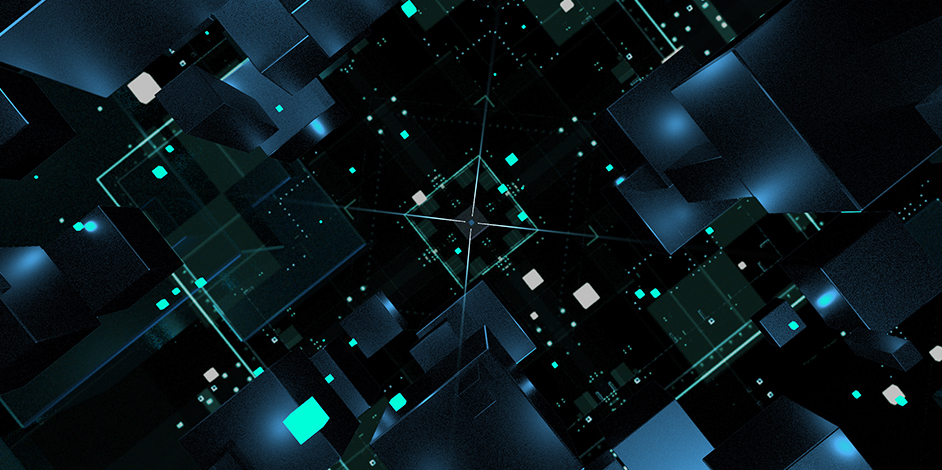
08 / 09
DRL algorithm framework design
Aiming at the calculation models of various deep reinforcement learning algorithms, design and abstract general DRL programming models, combining multiple decision-making and planning methods to build hybrid AI, which can be easily applied to multiple environments and multiple computing scales, thereby enhancing generalization and ease of use.
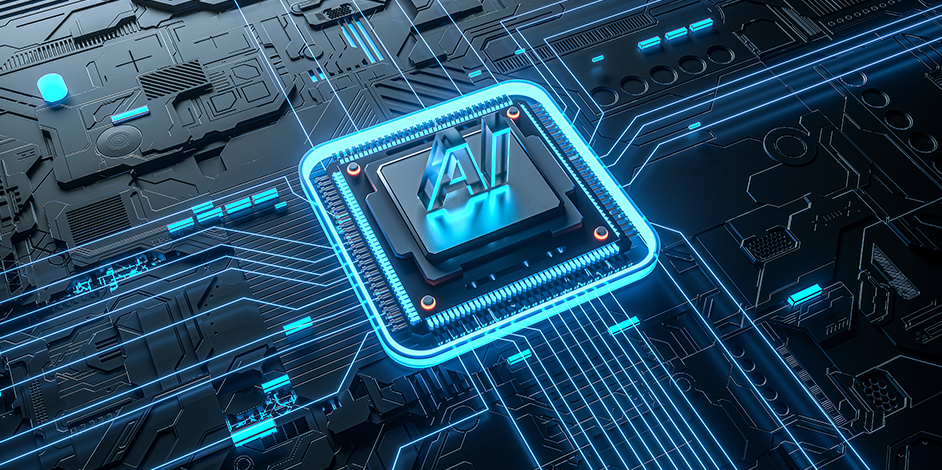
09 / 09
Distributed DRL system design and optimization
Combining the characteristics of AI algorithms and system design methods to improve the data throughput and resource utilization of distributed reinforcement learning training, design an efficient and stable distributed communication system, and specifically optimize core performance bottlenecks such as data transmission and model transmission, and build a general infrastructure that can support various applications and related benchmark test sets. Finally, create more forms for AI+System.
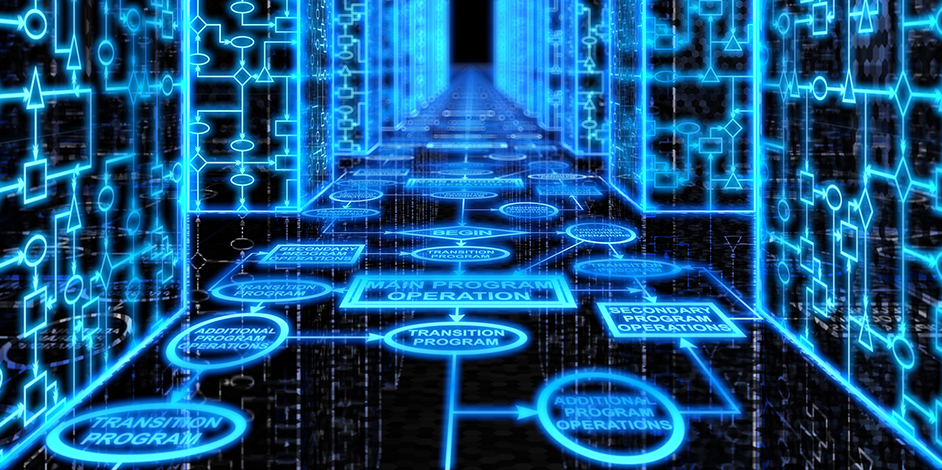
- Game Agent
- League Learning
- DI-zoo Decision AI algorithm Zoo
- Autonomous Driving Decision System
- Traffic signal control
- Complex computational graph optimization
- Multimodal data processing
- DRL algorithm framework design
- Distributed DRL system design and optimization
01 / 09
Game Agent
We support artificial intelligence agents capable of accompanying, taking over offline players, high-level competing in real-time strategy, frist person shooting and sports games.
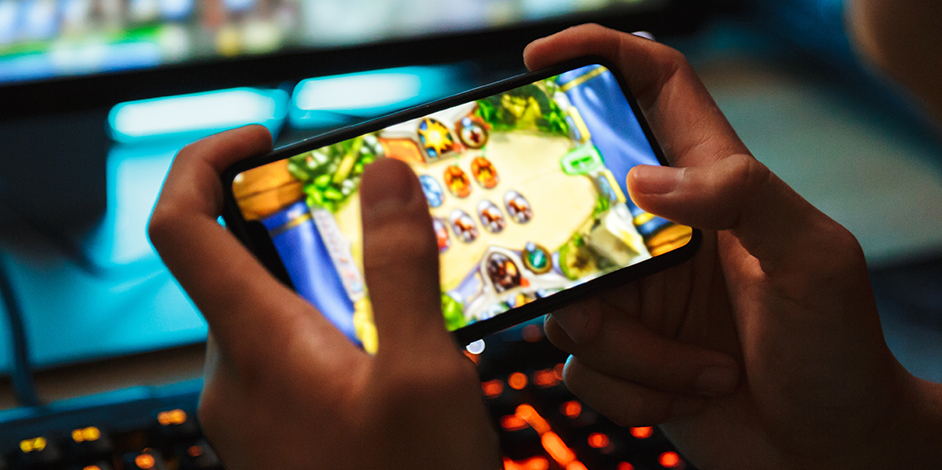
02 / 09
League Learning
Large-scale reinforcement learning framework. Starting from self-play, a single agent can expand to a league with hundreds of agents during training. This framework produces agents in different levels through well-designed match mechanism and massive games.
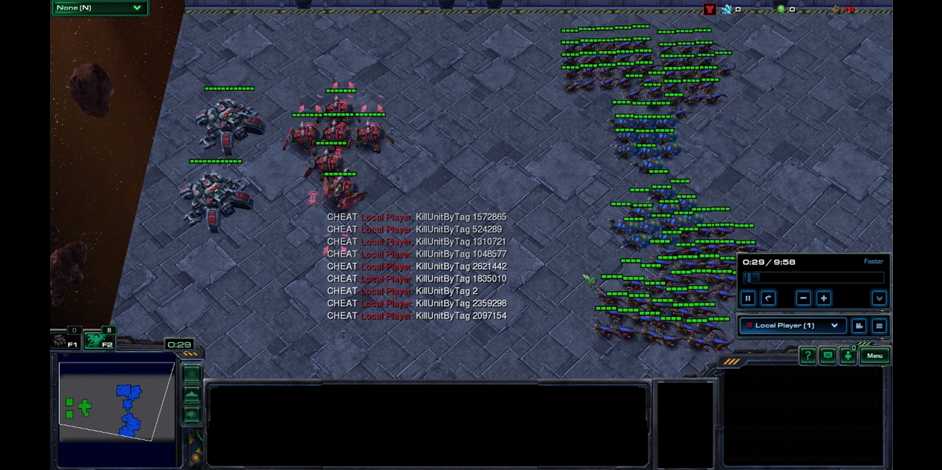
03 / 09
DI-zoo Decision AI algorithm Zoo
DI-zoo provides abundant implementations of reinforcement learning(RL) algorithms. It features well-tuned training configurations, efficient implementations and a pretrained algorithm zoo. We aim at helping researchers and engineers to quickly start learning RL, validate their ideas and easily get baselines for their custom environments.
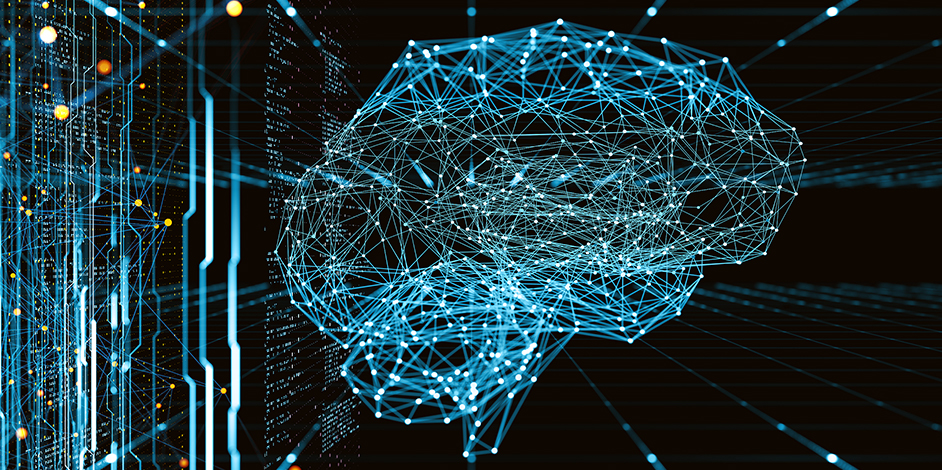
04 / 09
Autonomous Driving Decision System
Build an essential bridge between Decision AI and Autonomous Driving. This system helps to apply advanced Decision AI algorithms in academia to auto-driving tasks, and conduct training as well as evaluation with real vehicle data.
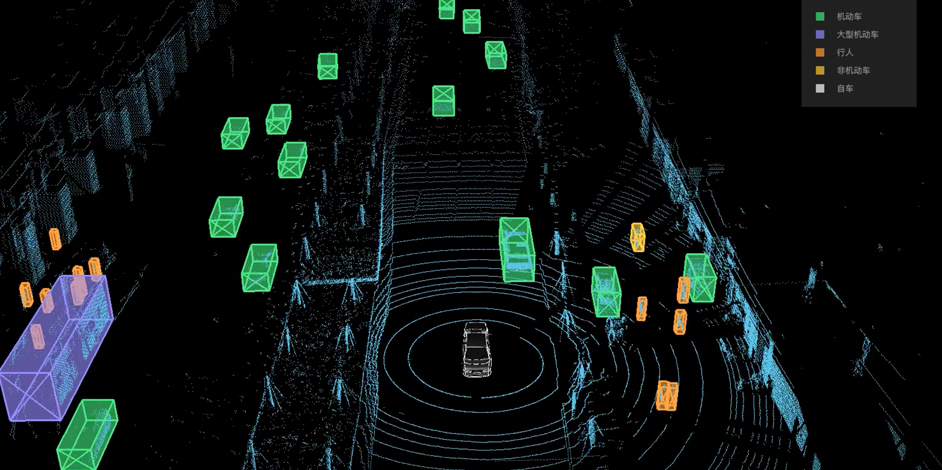
05 / 09
Traffic signal control
The Decision Intelligence technology is applied to city-level traffic signal light control, tidal lane change, etc., to maximize transportation efficiency. The actual effect on-road tests is 8%~47% higher than that of the traditional signal control methods.
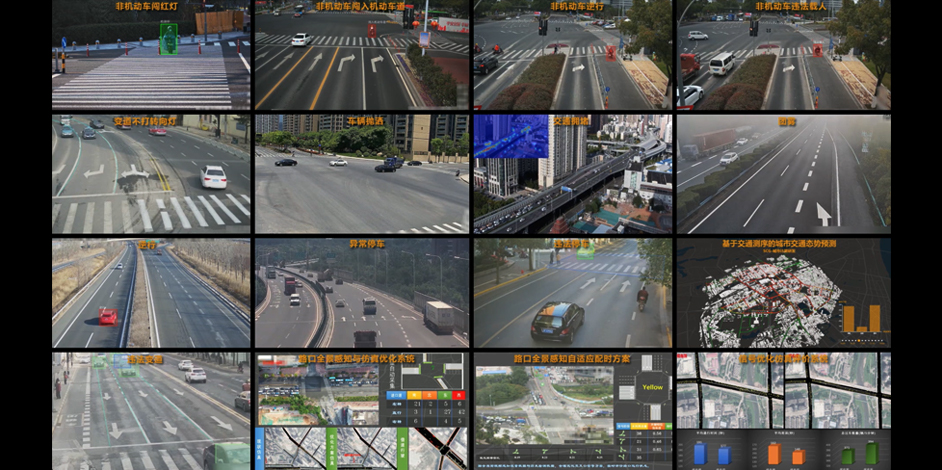
06 / 09
Complex computational graph optimization
According to the data flow optimization, design of lightweight computational graph and stable optimization of large-scale model training, it realizes the training optimization of the complex computational graph with high performance and low resource cost.
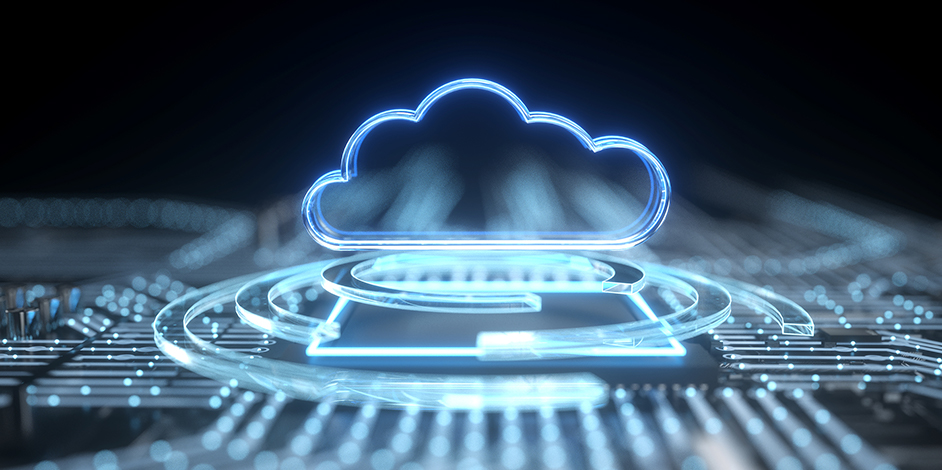
07 / 09
Multimodal data processing
According to the design of complex information extraction architecture for different modal data, it quickly realizes the effective feature extraction and efficient information aggregation of multiple modal data, and empowers model optimization based on multi-modal information.
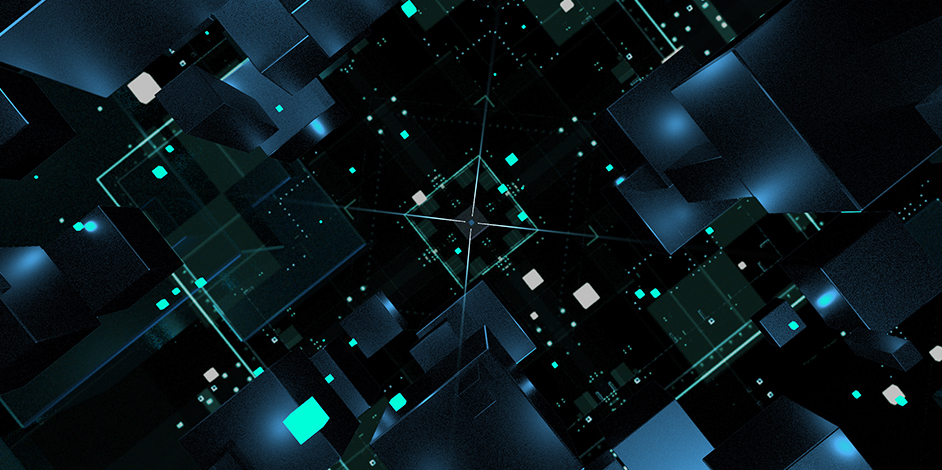
08 / 09
DRL algorithm framework design
Aiming at the calculation models of various deep reinforcement learning algorithms, design and abstract general DRL programming models, combining multiple decision-making and planning methods to build hybrid AI, which can be easily applied to multiple environments and multiple computing scales, thereby enhancing generalization and ease of use.
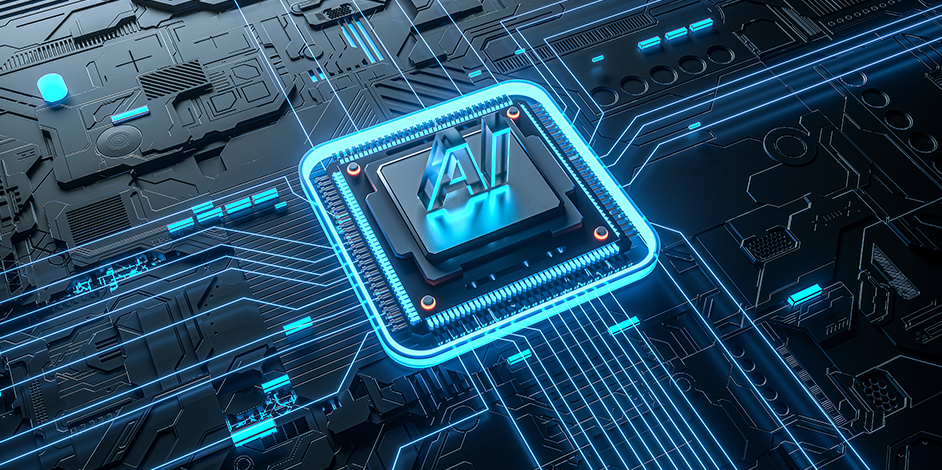
09 / 09
Distributed DRL system design and optimization
Combining the characteristics of AI algorithms and system design methods to improve the data throughput and resource utilization of distributed reinforcement learning training, design an efficient and stable distributed communication system, and specifically optimize core performance bottlenecks such as data transmission and model transmission, and build a general infrastructure that can support various applications and related benchmark test sets. Finally, create more forms for AI+System.
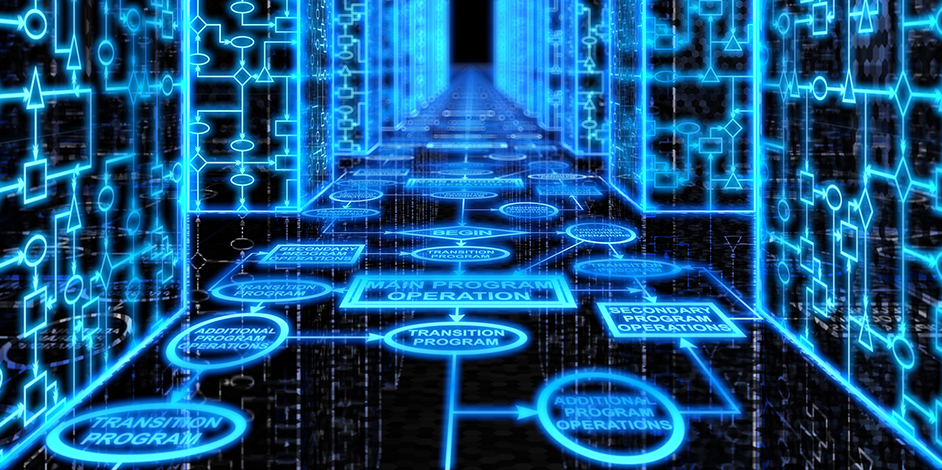
Partnership
Apply for Trial
Fill out this short form, and we’ll get back to you as soon as possible.
Apply for TrialBusiness Partnership
Email: business@sensetime.com
You haven't completed the information
After completing the information,
you can download the information